Join Rainbird Community
Enter the world of Rainbird and experience how Trustworthy AI can transform your Decision Intelligence.
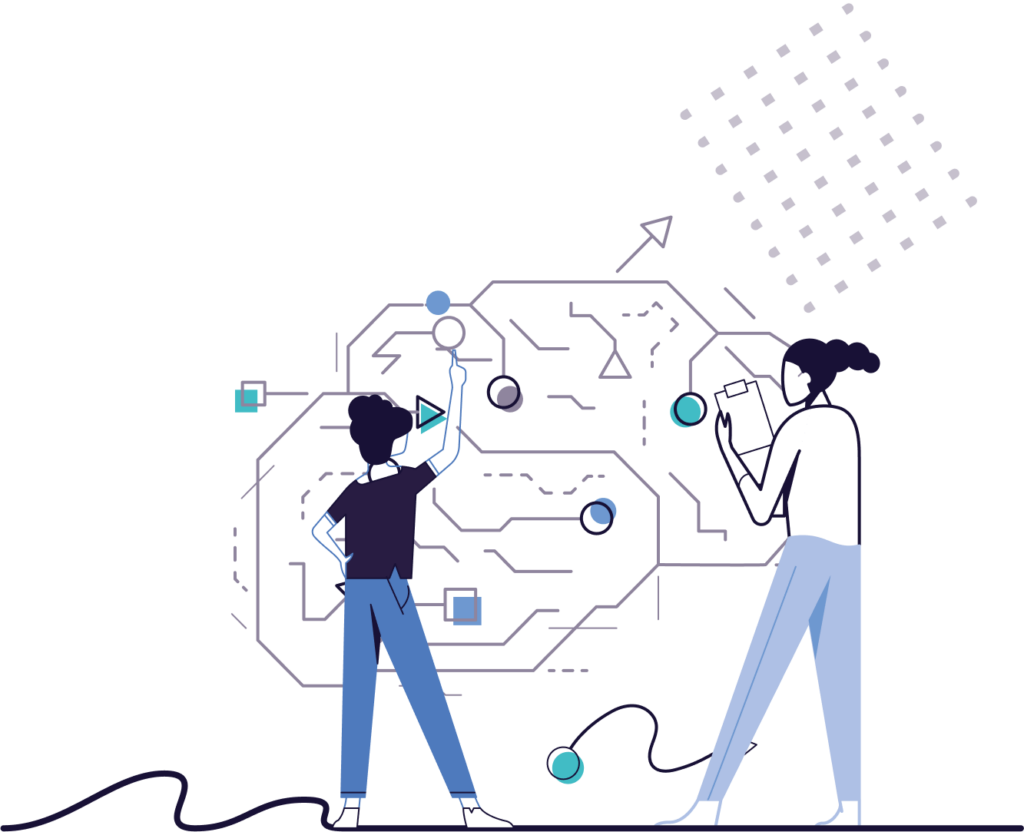
Why join?
By joining the Rainbird community, you can access free tools to build and deploy explainable AI models for complex decision-making. Our community provides valuable learning resources and platform access so you can gain hands-on experience with our technology.
How to get started
1. Enrol in our Academy and choose a learning path tailored to your goals.
2. Sign up for free access to our Community Edition platform. Follow along with hands-on build tutorials as you learn.
3. Contact us if you need additional support or to schedule a demo. We’re happy to help you get started.
Academy
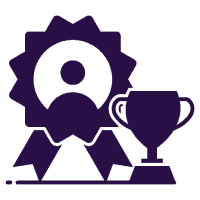
Learn to visually encode human expertise and data into extended knowledge graphs to make complex, automated decisions.
Unlock the full potential of decision intelligence with transparency and explainability baked in.
Community
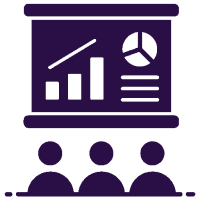
Get free access to our Community platform to automate your own decisions and scale your expertise.
Join us now and start building AI models that are traceable, reliable and free from bias.
Resources
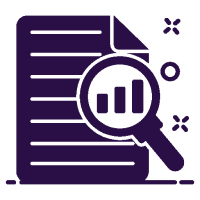
Additional resources to aid you in your journey to delivering explainable AI.
Support
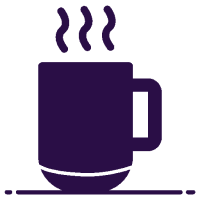
Get in touch with our team of experts if you have any questions, queries, or suggestions – they will be happy to help!
Transform your business into a Decision Intelligence powerhouse
Explore how Rainbird can seamlessly integrate human expertise into every decision-making process. Embrace the future of Decision Intelligence powered by explainable AI.